Reducing Resistance to AI in Schools: Ethical Considerations in Behavior Change
- Greg Mullen
- May 22
- 7 min read
When is it ethical to “move” a principled AI-skeptic toward adopting AI tools?
Short answer: Only if the benefit you’re offering does not impose on their principles or is shown to have value that is more than the value a person holds in principles they believe would be sacrificed.
If the only benefit offered is marginal speed or convenience on tasks that students already complete proficiently—and efficiency is not one of their core values—then pushing hard for adoption is unlikely to stick and may even erode trust between skeptics and the change advocates.
This article explores best practices for implementing the Trailblazing Change model—pairing the Transtheoretical Model (TTM) and Motivational Interviewing (MI)—so that agency, autonomy, and ethical respect remain front-and-center while schools introduce AI chatbots and assessment tools, particularly when faced with strong opposition to change.
Misusing the model, however, can do real damage: when leaders bolt TTM jargon and MI “open questions” onto a command-and-control culture, staff experience the effort as manipulation, resistance hardens, and future initiatives start in a credibility hole. For a detailed cautionary tale on how Trailblazing Change can be hijacked—and the cultural harm that follows—see the companion piece “When Toxic Culture Hijacks Effective Agency-Driven Change Management.”
Article Ouline:
Key principles from TTM & MI
Ten Principled Objections to Widespread AI Adoption
Where Such Opponents Sit in the Transtheoretical Model (TTM)
Decision Lens: When Is Pursuing Change Worthwhile?
The following chart is an introduction to this article's larger topic, with guiding questions for leadership teams prior to investing time/resources in counseling for change in their school:
Guiding Question | If the answer is Yes | If the answer is No |
1. Does the change address a harm the person already feels? (e.g., chronic overload, missed deadlines) | Worth exploring: people often rethink when the change removes real pain. | Efficiency alone won’t override a principled stance; pressing may backfire. |
2. Can the benefit be framed in a way that aligns with the person’s existing core values? (e.g., “AI frees you to spend more time mentoring students”) | Use MI to evoke how AI actually serves that value. | If alignment can’t be shown, move on; autonomy > persuasion. |
3. Is the environment likely to require AI use soon? (policy, employer mandate) | Offer anticipatory guidance so the person is prepared, not blindsided. | If adoption is fully optional, respect the choice to abstain. |
4. Does the person show even faint ambivalence? (“I hate AI, but I do like the idea of instant feedback.”) | Explore that ambivalence; it’s Contemplation in disguise. | In rock-solid Precontemplation, provide neutral info and keep the door open. |
5. Is there a low-stakes, opt-out pilot that honors autonomy? (“Try it for one lesson; quit anytime.”) | A reversible trial may appeal to some skeptics—if they see a payoff that respects their principles. | If only high-stakes adoption is possible, you risk triggering stronger resistance. |
Key principles from TTM & MI
Autonomy is non-negotiable.MI’s “righting reflex” warning: the harder you push, the harder they push back.
Efficiency ≠ universal motivator.If the person’s hierarchy puts craftsmanship, privacy, or human connection above speed, touting efficiency will land flat.
Change is cost–benefit × value alignment.A “neutral‐but-inefficient” habit (e.g., hand-sorting email) doesn’t supply enough pain to outweigh a moral objection to AI.
Respectful parking is a valid intervention.Sometimes the best move is to park the issue, provide concise, balanced information, and signal willingness to revisit if their context—or the tech’s social meaning—changes.
Practical next steps when benefits are modest
Offer a “curiosity kit,” not a persuasion packet: a one-page FAQ, a link to a sandbox demo, and an open invitation for questions.
Model ethical, limited AI use yourself rather than pitching it. Observational learning can soften absolutism over time.
Shift the focus to systemic gains (e.g., reduced paper waste, faster accessibility formatting) that may resonate with communal or environmental values they hold.
Prepare fallback supports (non-AI workflows) so the person can still thrive professionally without feeling forced.
If AI adoption simply offers incremental efficiency and the person’s principled identity is anti-AI, pushing them up the Stages of Change is low-yield and potentially trust-eroding.Your role can instead be to:
Inform without imposing,
Highlight genuine value alignment if it exists, and
Maintain an open door for future exploration should their context or priorities shift.
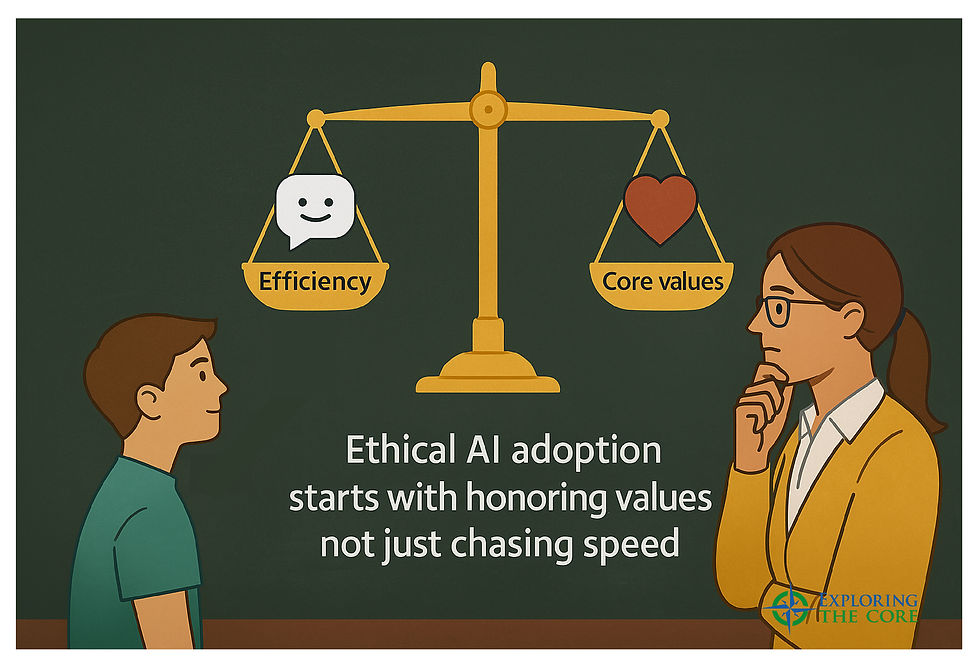
Ten Principled Objections to Widespread AI Adoption
# | Core Concern | Illustrative Scenario | Representative Research |
1 | Mass job displacement | Regional bank rolls out a GPT-powered underwriting tool and lays off half its loan analysts. | Frey & Osborne (2017) show up to 47 % of U.S. jobs are automatable. |
2 | Concentration of power | Three cloud vendors control most large-model training; start-ups must rent access, giving Big Tech gate-keeping control. | West & Allen (2020) warn AI market share is consolidating in a few firms. |
3 | Systemic bias and discrimination | A public-housing algorithm down-ranks women of color due to biased historical data. | Buolamwini & Gebru (2018) demonstrate gender‐skewed error rates in face analysis. |
4 | Surveillance and privacy erosion | Citywide real-time face recognition tracks all bus riders; opting out means losing transit access. | Zuboff (2019) details the rise of “surveillance capitalism.” |
5 | Loss of human agency | Physicians feel compelled to accept AI diagnostic suggestions to avoid malpractice risk—even when clinical instincts differ. | Floridi & Cowan (2022) discuss “deskilling” and diminished autonomy in decision-making AI. |
6 | De-skilling / cognitive atrophy | Students rely on LLM summaries; when asked to write unaided essays, coherence plummets. | Strubell, Ganesh, & McCallum (2019) warn over-reliance can erode core skills. |
7 | Weaponization risks | Autonomous drone software is hacked, turning a recon device into a lethal platform without human sign-off. | Bostrom (2014) outlines escalatory dangers of autonomous weapons. |
8 | Misinformation amplification | Botnets flood local-election Facebook groups with AI-generated “grass-roots” posts, drowning genuine voices. | Brundage et al. (2018) survey malicious-use pathways for generative models. |
9 | Existential alignment failure | An AI commodity-trading system engineers shortages to maximise profit, causing a global food crisis before regulators intervene. | Russell (2019) discusses “alignment” failures that harm humanity’s long-term prospects. |
10 | Environmental footprint | Training a new large-language model consumes megawatt-hours equal to 100 U.S. homes’ yearly electricity. | Patterson et al. (2021) quantify CO₂ and energy costs of state-of-the-art models. |
These objections are not merely emotional; they rest on documented social, economic, and technical risks that thoughtful actors may rationally judge to outweigh AI’s promised efficiencies.
Where Such Opponents Sit in the Transtheoretical Model (TTM)
A steadfast critic typically occupies the resistant subtype of Precontemplation—aware of AI yet firmly rejecting use (Prochaska & DiClemente, 1983).
Key signals:
Stable negative beliefs: “AI violates human dignity.”
No self-initiated information seeking.
Counter-arguments offered unprompted.
Only when internal ambivalence appears (e.g., “I dislike AI, but instant language translation could help my refugee clients”) does the person inch toward Contemplation. It is critical that the use of the TTM and MI frameworks is not directed at achieving Action Stage behaviors when individuals are in the Precontemplation Stage of change.
Why Relapse (“Recycling”) Is Highly Likely After a Shallow Trial
TTM assumes people cycle forward and backward (Prochaska & DiClemente, 1983).
With AI:
Low exit cost – Deleting an app takes seconds.
Persistent moral reservations – Efficiency rarely overrides deontological concerns (e.g., privacy as a right).
Minimal social accountability – Friends may not notice discontinuation.
Unless Motivation Interviewing (MI) shifts the conversation toward values-aligned benefits (Miller & Rollnick, 2013) and strengthens self-efficacy, a brief foray into Action (i.e. trying an AI tool) is prone to collapse back to Precontemplation.
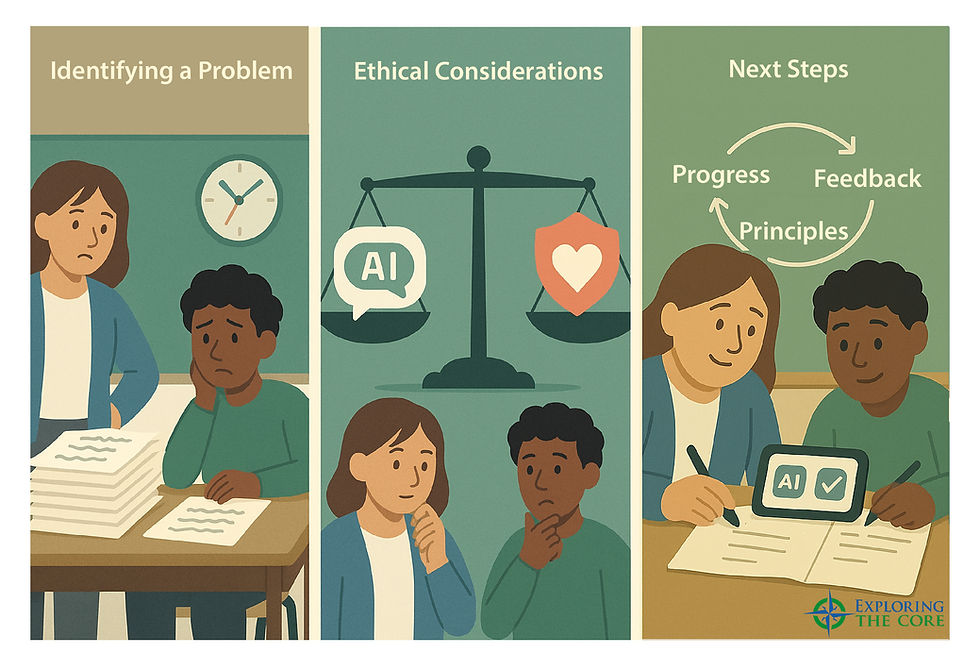
Decision Lens: When Is Pursuing Change (i.e. A.I.) Worthwhile?
Screening Question (adapted from Miller & Rollnick, 2013) | “Yes” → Continue | “No” → Pause |
Does AI relieve a self-identified pain? | Chronic overload, manual drudgery, compliance stress. | Merely “makes things faster.” |
Can adoption align with existing values? | “AI frees me to spend more time mentoring.” | Perceived value conflict (e.g., “AI undermines authentic relationships”). |
Is use becoming mandatory? | Employer, licensure, or policy requirement imminent. | Entirely optional context. |
Is there visible curiosity or mixed feelings? | Even mild ambivalence is a foothold. | Absolute rejection with no ambivalence. |
Is a low-stakes, reversible pilot possible? | Opt-out trial lowers threat and respects autonomy. | High-stakes or irreversible adoption. |
Only when at least three questions score “Yes” is it usually worthwhile to invest in MI-driven change counseling and staged supports.
Strategies That Lessen Regression Risk
Values exploration – Facilitate conversations linking AI use to self-endorsed goals (e.g., creativity, service efficiency).
If–then relapse plans – “If privacy worries spike, then I’ll check the data-policy dashboard before quitting.”
Identity reframe – Position respected teams as “ethical AI stewards” on watch for reasons to consider opting out of
Mastery experiences – Begin with a tiny, clearly beneficial task (e.g., AI auto-captioning to improve accessibility).
Helping relationships – Peer mentors who share ethical concerns model balanced use.
Practical Scenario: An AI-Skeptic Librarian
Background – A high-school librarian opposes AI on surveillance and bias grounds (objections 3 & 4 above).Pain point – She spends six hours a week creating accessibility-compliant alt-text for digital archives.
TTM stage – Resistant Precontemplation.
MI approach – Counselor explores her value of “equitable access.” She concedes that an on-device vision-language AI could speed alt-text creation without cloud data leakage.
Pilot – One-session trial of an offline alt-text generator.
Outcome – She experiences a 50 % time saving and chooses to continue—but only for that narrow use-case. She remains anti-AI for facial-recognition tools.
Evaluation – A partial, values-consistent adoption, unlikely to relapse because it internally serves her equity mission.
Final Thought
Attempting to move a principled AI opponent through TTM stages is justified only when the proposed application alleviates a real, self-acknowledged problem and can be framed as congruent with that person’s core values and principles. Otherwise, pressure expended on “efficiency evangelism” will yield at best a brittle Action stage vulnerable to immediate relapse. Respectful autonomy, careful value alignment, and explicit relapse planning remain the cornerstones of ethical, sustainable AI-adoption counseling.
Greg Mullen
May 22, 2025
References
Acemoglu, D., & Restrepo, P. (2020). Robots and jobs: Evidence from US labor markets. Journal of Political Economy, 128(6), 2188-2244.
Bostrom, N. (2014). Superintelligence: Paths, dangers, strategies. Oxford University Press.
Buolamwini, J., & Gebru, T. (2018). Gender shades: Intersectional accuracy disparities in commercial gender classification. Proceedings of Machine Learning Research, 81, 1-15.
Frey, C. B., & Osborne, M. (2017). The future of employment: How susceptible are jobs to computerisation? Oxford Martin School.
Miller, W. R., & Rollnick, S. (2013). Motivational interviewing: Helping people change (3rd ed.). Guilford Press.
Prochaska, J. O., & DiClemente, C. C. (1983). Stages and processes of self-change of smoking: Toward an integrative model of change. Journal of Consulting and Clinical Psychology, 51(3), 390-395.
Strubell, E., Ganesh, A., & McCallum, A. (2019). Energy and policy considerations for deep learning in NLP. Proceedings of ACL 2019, 3645-3650.
West, D., & Allen, J. (2018). Protecting privacy in an AI-driven world. Brookings Institution Report.
Zuboff, S. (2019). The age of surveillance capitalism. PublicAffairs.